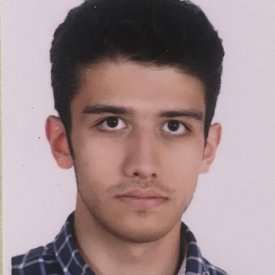
حسین حاجیانی
تبیین داده های پتانسیل رویدادوابسته ی عصب زبانشناختی با استفاده از مدل های زبانی بزرگ
- دانشجو
- حسین حاجیانی
- استاد راهنما
- صغرا ابراهیمی قوام
- استاد مشاور
- محمد بحرانی
- استاد داور
- حسن اسدزاده هرائی
- مقطع تحصیلی
- کارشناسی ارشد
- محل دفاع
- روانشناسی و علوم تربیتی
- شماره ساختمان محل ارائه
- ۳۱
- نام کلاس محل ارائه
- کلاس ۱۵[۲۲۱۵]
- شماره کلاس محل ارائه
- ۱۱۵
- تاریخ دفاع
- ۲۸ شهریور ۱۴۰۳
- ساعت دفاع
- ۰۸:۰۰
- چکیده
-
یکی از انواع دادههای عصبی که تا کنون به عنوان شاخص پیشبینی زبانی توسط مغز به کار رفتهاند، مؤلفههای رویدادوابسته و در رأس آنها مؤلفهی N۴۰۰ است. پژوهشها رابطهی مؤلفهی N۴۰۰ را با پیشبینیپذیری و معقول بودن کلمات در زمینه نشان دادهاند. با این وجود هنوز راجع به نحوهی دقیق ارتباط این مؤلفه با پیشبینی، اختلاف نظر وجود دارد. در یک سوی طیف، رویکرد متضاد با پیشبینی قرار دارد که N۴۰۰ را صرفاً نتیجهی تلاش مغز برای یکپارچهسازی کلمه با زمینه میداند و در سوی دیگر، رویکرد پیشبینی حداکثری را داریم که قائل به تسری پیشبینی زبانی حتی تا سطح واجشناختی است. یافتههای یکی از پژوهشهای مهم N۴۰۰ در این زمینه حاکی از پیشبینی حروف تعریف نکرهی انگلیسی - که مستقیماً بر اساس ویژگیهای واجشناختی کلمهی پس از خود انتخاب میشوند - توسط مغز است. این مشاهده که در گذشته به عنوان دلیلی محکم بر صحت پیشبینی حداکثری به شمار میآمد، بعدها توسط یک پژوهش دیگر روی نمونهای به مراتب بزرگتر زیر سؤال رفت. متغیر مستقل در هر دو پژوهش، یعنی پیشبینیپذیری حروف تعریف انگلیسی، با احتمال کلوز این کلمات اندازهگیری شدهاست؛ شاخصی که بر اساس جمعآوری پرسشنامه از نمونههای نسبتاً کوچک محاسبه میشود و از این رو روایی و پایایی آن برای سنجش میزان پیشبینیپذیری کلمات مورد تردید است. یک روش جایگزین که اخیراً پیشنهاد شده، استفاده از پیشبینی مدلهای زبانی بزرگ راجع به کلمات (مقدار سورپرایزال آنها برای هر کلمه) است؛ مدلهایی مبتنی بر شبکهی عصبی ترنسفورمر، که با پیمایش پیکرههایی به حجم صدها میلیارد کلمه آموختهاند کلمهی بعدی متن را پیشبینی کنند. در این پژوهش ما مجدداً شواهد N۴۰۰ مبنی بر پیشبینی واجشناختی حروف تعریف نکرهی انگلیسی را بررسی کردیم و نشان دادیم که میتوان با استفاده از سورپرایزال مدلهای زبانی بزرگ به جای احتمال کلوز، اثر N۴۰۰ را علاوه بر پیشبینی اسمها، برای پیشبینی این گروه از کلمات نیز مشاهده کرد.
- Abstract
-
One type of neural data that has so far been used as an index of language prediction by the brain is event-related components, with the N۴۰۰ component being the most prominent. Research has shown the relationship between the N۴۰۰ component and the predictability and plausibility of words in context. However, there is still disagreement about the exact nature of this relationship with prediction. On one end of the spectrum, there is an anti-prediction approach, which views N۴۰۰ merely as the brain's effort to integrate a word with the context. On the other end, there is the maximal prediction approach, which argues for the extension of language prediction even to the phonological level. Findings from one important study on N۴۰۰ in this area suggested that the brain predicts the indefinite articles in English—selected directly based on the phonological features of the following word. This observation, which was previously considered strong evidence for maximal prediction, was later questioned by another study conducted on a much larger sample. The independent variable in both studies, i.e., the predictability of English articles, was measured using the cloze probability of these words—a measure calculated based on questionnaires collected from relatively small samples, raising doubts about its validity and reliability for assessing word predictability. A recently proposed alternative method is to use the predictions of large language models regarding words (i.e., the surprisal value for each word). These models, based on transformer neural networks, have been trained to predict the next word in a text by analyzing corpora containing hundreds of billions of words. In this study, we reexamined the N۴۰۰ evidence for the phonological prediction of English indefinite articles and demonstrated that, by using the surprisal values from large language models instead of cloze probability, the N۴۰۰ effect can be observed not only for nouns but also for the articles preceding them.